Covariance and correlation are fundamental statistical measures that play a significant role in various fields, yet they often remain misunderstood. To fully comprehend their significance, it is essential to grasp the underlying approach and the reasons behind their widespread usage in finance.
One most important aspect of an investment portfolio is the diversification benefit which is captured by the correlation coefficient- to measure the amount of diversification among the assets held in the portfolio. And for that, we have to determine the covariance- which measures the directional relationship between each pair of stocks in a portfolio.
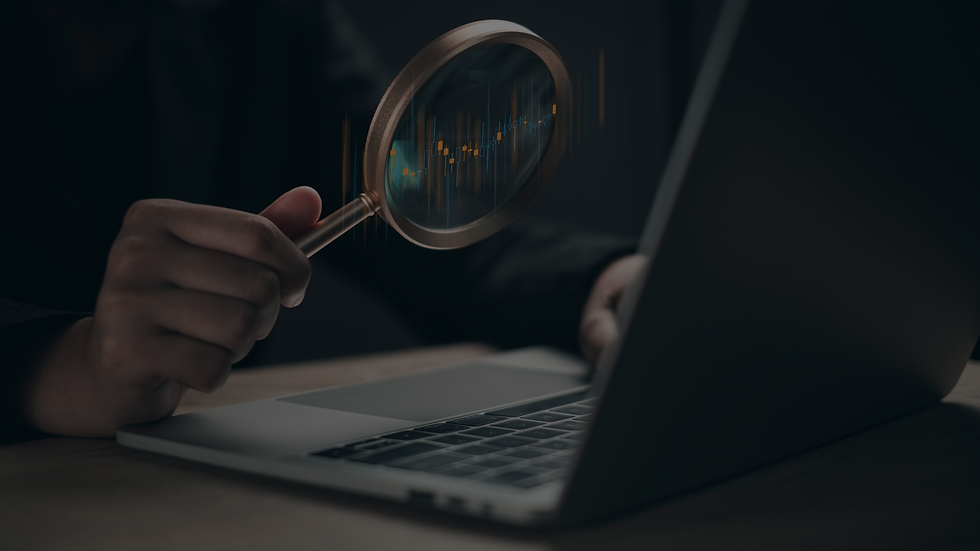
More formally, covariance is a statistical measure that describes the directional relationship between two random variables (whether they are positively or negatively correlated, but linear relationship) and correlation is a statistical measure that quantifies the direction and strength of linear association between those variables (ranges from -1 to +1).
Pearson's Correlation Coefficient Classification
Pearson's correlation coefficient, also known as Pearson's r, is a widely used measure of the strength and direction of the linear relationship between two variables. It ranges between -1 and 1, where:
A correlation coefficient of 1 indicates a perfect positive linear relationship. This means that as one variable increases, the other variable also increases proportionally.
A correlation coefficient of -1 indicates a perfect negative linear relationship. In this case, as one variable increases, the other variable decreases proportionally.
A correlation coefficient close to 0 suggests no linear relationship between the variables. The variables may be independent or related through a nonlinear pattern.
Based on the magnitude of the correlation coefficient, correlations are often classified as follows:
Strong positive correlation: A correlation coefficient close to 1 indicates a strong positive linear relationship. This means that the variables tend to move closely together in a positive direction.
Strong negative correlation: A correlation coefficient close to -1 indicates a strong negative linear relationship. The variables tend to move closely together but in opposite directions.
Weak positive correlation: A correlation coefficient between 0 and 0.5 (exclusive) suggests a weak positive linear relationship. The variables show some tendency to move together in a positive direction, but the relationship is not very strong.
Weak negative correlation: A correlation coefficient between 0 and -0.5 (exclusive) suggests a weak negative linear relationship. The variables show some tendency to move together but in opposite directions, although the relationship is not very strong.
No correlation: A correlation coefficient close to 0 indicates no linear relationship between the variables.
It's important to note that correlation coefficients measure only linear relationships and may not capture complex or nonlinear associations between variables. Additionally, correlation does not imply causation, meaning that a strong correlation between two variables does not necessarily indicate a cause-and-effect relationship between them.
Correlation Coefficient | Classification | Classification |
r = +1 | Perfect Positive | Perfect Positive Linear Relationship |
+0.5 < r < +1 | Strong Positive | Strong Positive Linear Relationship |
0 < r < +0.5 | Weak Positive | Weak Positive Linear Relationship |
r = 0 | No Correlation | No Linear Relationship |
-0.5 < r < 0 | Weak Negative | Weak Negative Linear Relationship |
-1 < r < -0.5 | Strong Negative | Strong Negative Linear Relationship |
r = -1 | Perfect Negative | Perfect Negative Linear Relationship |
For example, a portfolio comprises two stocks- X and Y (called variables). Each variable has its pase (volatility) at which it is changing every moment. If both variables tend to move in the same direction, it is said that they are positively correlated. And the rate at which they are moving in the same direction is identified by correlation.
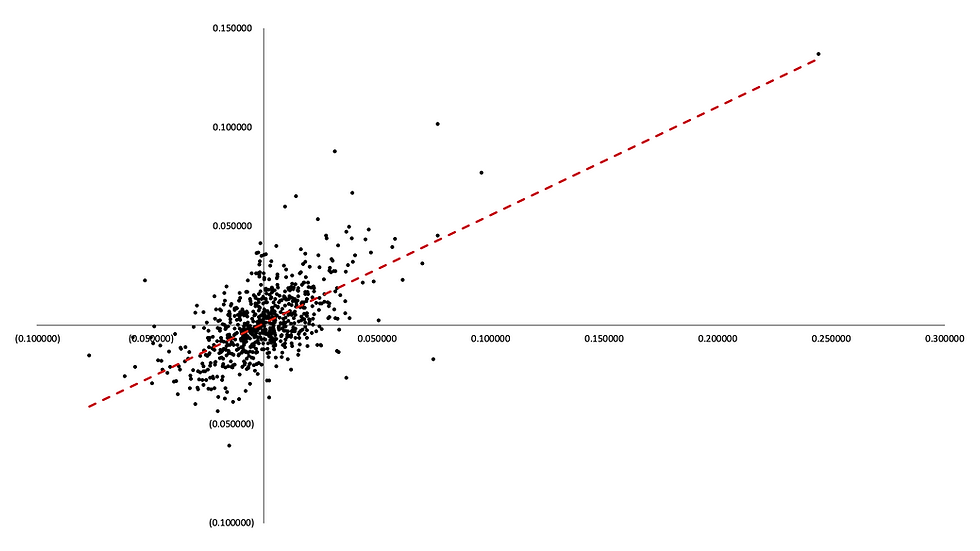
Variance and Covariance are the fundamental measures of risk and the relationship between two variables, respectively. While Standard Deviation and Correlation are the standardized approaches calculated for the purpose of convenience.
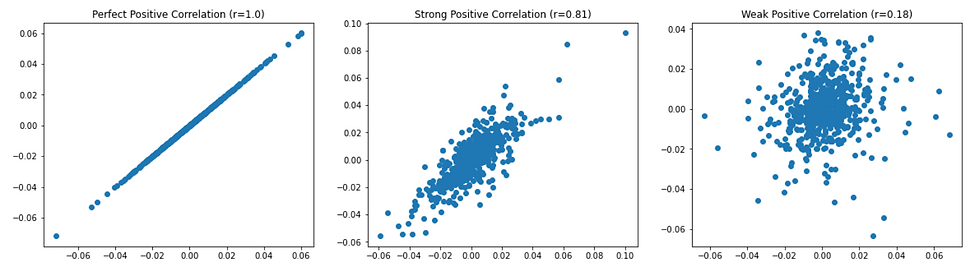
Let's understand Covariance & Correlation using market data-
Refer to our Introductory article on Construction of Optimal Portfolio - which explained in detail how to extract the data from Yahoo Finance and calculate the daily continuous return and deviation from its mean return for all five stocks.
The correlation coefficient acts as an ingredient in the Construction of an Optimal Portfolio. The reason is risk reduction benefits arising out of diversification. The process of calculating the correlations is straightforward-
Step - 01: Calculate the Squared Returns Deviations for individual stocks-
Take the square root of the individual return deviations from its mean return (i.e., √ (Return - Mean Return)) calculated in the referred article to capture both upside and downside fluctuation in the stock price using the Matrix Multiplication Function in Excel as shown below-
{=MMULT(TRANSPOSE(return matrix), return matrix)}

Step - 02: Calculate the Covariance and Correlation for individual stocks-
One most important aspect of portfolio management is the diversification benefit which is captured by the correlation coefficient- to measure the amount of diversification among the assets contained in a portfolio. And for that, we have to identify the covariance- which measures the directional relationship between each pair of stocks in our portfolio (nothing but the sum product of mean deviation of ith stock with a mean deviation of jth stock) as shown below-

=Squared Deviation of Returns/N-1

=Covariance/MMULT(TRANSPOSE(standard deviation), standard deviation)
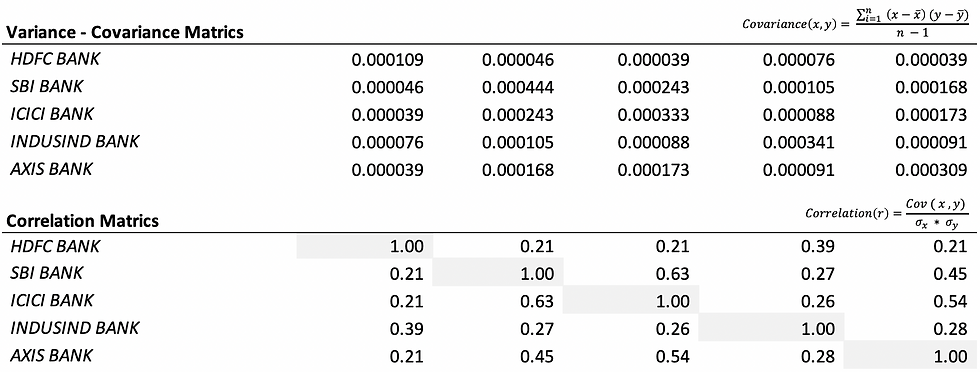
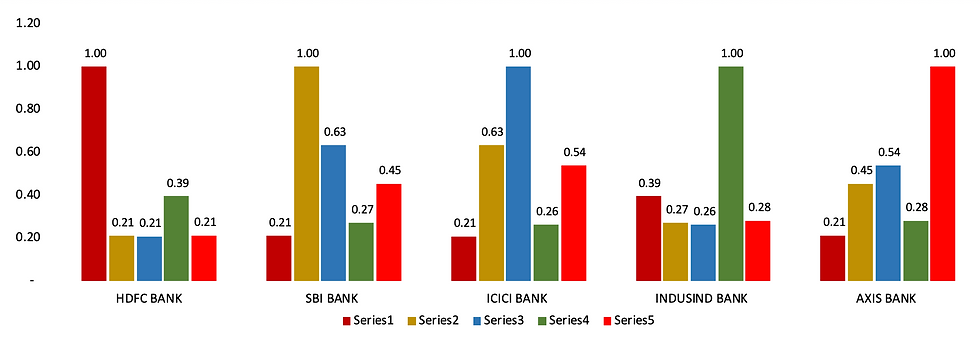
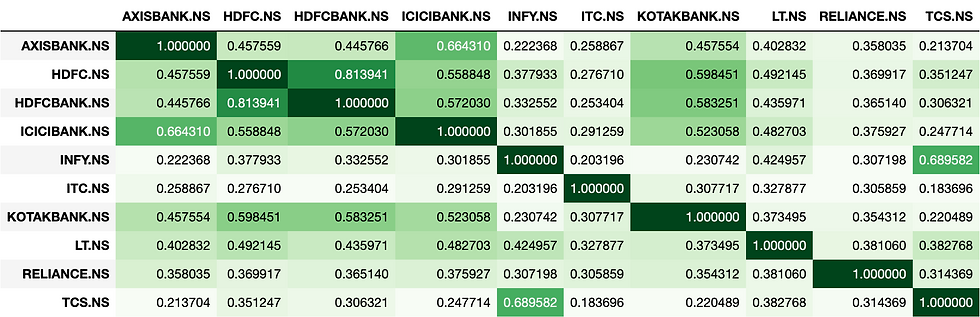
If you are still wondering- Why standardization is required? if we have covariance!
-- The problem with the covariance is that it keeps the scale of co-movement of both the variables X and Y with no threshold on the levels. And that makes it difficult to interpret and impossible to make comparisons with others. It only tells us whether these pairs are positively or negatively correlated, but it is difficult to tell whether the relationship between X and Y is stronger or weaker than P and Q. This is where correlation comes in — by standardizing covariance by some statistical measure of variability in the data, it produces a quantity that has intuitive interpretations and a consistent scale.
Comentários