Value-at-Risk (VaR) for Risk Professionals: Methodologies, Computation, and Practical Applications in Risk Management
- Pankaj Maheshwari
- Jan 1, 2025
- 5 min read
Updated: Mar 31
"What could be the maximum loss that can occur on my investments?" This is a question that every investor, trader, or risk manager asks at some point!
In the dynamic world of financial markets, quantifying potential losses is a foundational requirement for sound risk management. Among the various tools available to measure financial risk, Value-at-Risk (VaR) has emerged as one of the most widely adopted metrics. Alongside traditional measures such as variance and standard deviation, VaR provides an intuitive and probabilistic estimate of potential loss, which makes it highly practical and communicable—especially to senior management and regulators.
What is Value-at-Risk?
Value-at-Risk (VaR) is a statistical measure that quantifies the potential maximum loss an investment portfolio could experience at a certain confidence level over a specified time horizon. It provides a single risk number that helps traders, risk managers, and financial institutions assess how much they can potentially lose under normal market conditions.
In simpler terms, it answers:
"What is the worst-case loss I can expect with X% confidence over the next N days?"
for example, a 1-day VaR of $10 million at 99% confidence implies that there is a 99% chance that losses will be less than or equal to $10 million with a 1% chance that the portfolio could lose more than $10 million in a single day.
VaR is popular not only because of its conceptual clarity but also due to its broad applicability—from performance evaluation to internal risk control and regulatory capital determination.
A Brief History: VaR at J.P. Morgan
The widespread use of VaR began in the early 1990s when J.P. Morgan developed an internal system to measure the financial risk across its trading books. The approach involved:
Identifying key market risk factors, such as equity prices, interest rates, currency rates, and volatilities.
Constructing a variance-covariance matrix based on historical time series of returns.
Estimating the portfolio's exposure to each factor.
Calculating the portfolio VaR, incorporating the correlations between these risk factors to aggregate risk appropriately.
The system was designed to provide a single summary statistic, the VaR number, that could be reported across desks and business units, bringing consistency and discipline to the firm’s risk management practices.
In 1994, J.P. Morgan released this methodology to the public under the name RiskMetrics, which quickly became a standard in the industry and catalyzed the global adoption of VaR.
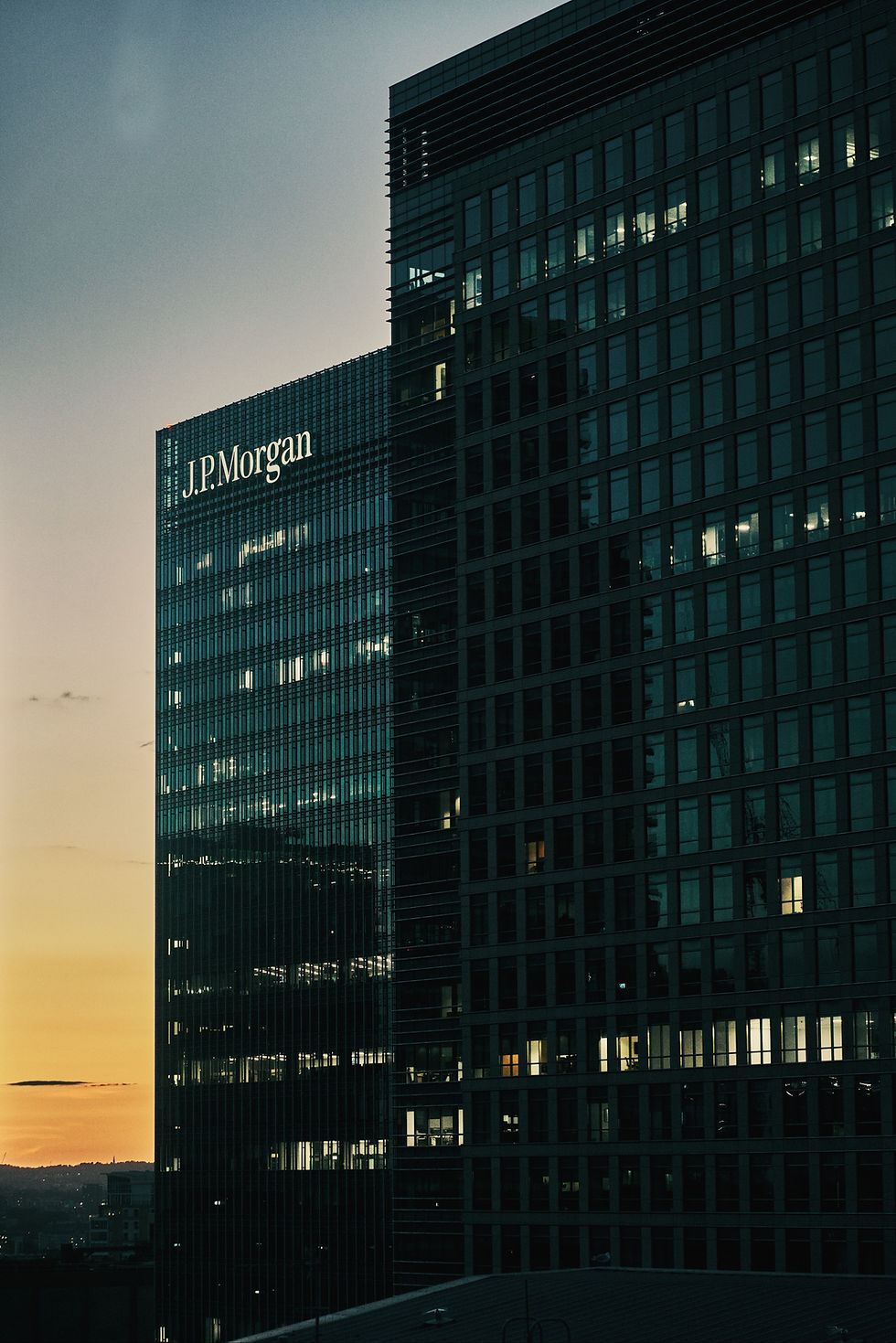
Since its inception, the Value-at-Risk framework has evolved significantly. It is now supported by a range of methodologies, including the Variance-Covariance Method (Parametric VaR), Historical Simulation, and Monte Carlo Simulation. Each method comes with its own set of assumptions and computational complexities.
Over the years, risk professionals and academics have proposed numerous enhancements to the basic VaR approach to address critical challenges such as non-normal return distributions, fat tails and skewness, time-varying volatility (GARCH models), liquidity and credit risk incorporation, and inadequacy in capturing extreme tail risk (leading to measures like Expected Shortfall).
Despite these developments, VaR remains a cornerstone of market risk assessment, particularly due to its intuitive appeal and regulatory acceptance under Basel II and Basel III frameworks.
In the coming sections of this series, we will break down VaR methodologies, implementation techniques, and real-world applications, covering:
Introduction to Value-at-Risk (VaR): Different Methodologies, Assumptions, and Limitations
This section will introduce the three major methodologies used to calculate Value-at-Risk, explaining the mechanics, formulas, and use cases for each approach:
Variance-Covariance (Parametric) Method: It assumes normally distributed returns and linear relationships; uses standard deviation, mean, and correlation across risk factors.
Historical Simulation: It uses historical return data to simulate potential future losses. No assumptions about return distribution are required.
Monte Carlo Simulation: It involves simulating thousands of random scenarios based on assumed distributions and risk factor behaviors.
VaR, though has some valid reasons for its popularity, especially within investment banks and other financial institutions, but it comes with a few limitations, such as lack of subadditivity, no information on tail severity, and dependence on data and distributional assumptions. These limitations have led to the development of coherent risk measures such as Conditional VaR (Expected Shortfall) and Stressed VaR, both of which address some of these shortcomings.
Assumptions and Limitations of Value-at-Risk (VaR) Methodologies: What Every Risk Manager Should Know
This section will critically analyze the underlying assumptions behind each VaR methodology and their impact on model accuracy and reliability.
Variance-Covariance (Parametric) Method: It assumes normal distribution of returns and constant volatility; struggles with fat tails, skewness, and non-linearity in portfolios.
Historical Simulation: It relies heavily on past data; performance is sensitive to the chosen lookback window and may fail to capture structural market changes or future events.
Monte Carlo Simulation: It requires accurate modeling of distributions and correlations; computationally intensive and subject to model risk.
This section will also explore limitations of VaR itself, such as its inability to measure tail risk beyond the confidence threshold, and introduce the concept of subadditivity and why VaR may fail as a coherent risk measure.
Value-at-Risk (VaR) for Individual Instruments vs. Multi-Asset Portfolios
This section will explore how VaR behaves when applied to:
Single Instruments (interest rate bond, equity stock, or derivative): It's easy to model but doesn’t account for diversification or interaction effects.
Multi-Asset Portfolios: This requires aggregation of risk across asset classes, accounting for correlations and covariances between instruments.
This section will also include portfolio VaR calculation using the variance-covariance matrix, risk aggregation and diversification benefits, and limitations when combining linear and non-linear assets (equities and options), and practical tools for risk managers to interpret and aggregate VaR in large, diversified portfolios.
Value-at-Risk (VaR) Interpretation, Application, and Use in Financial Risk Management
This section will address how VaR is used in practice by both risk professionals and regulators, including setting risk limits and capital allocations, daily monitoring of trading book risk, communicating risk to senior management using risk dashboards, and regulatory compliance (Basel II/III), internal models approval, and capital charge calculations.
The section will also discuss common pitfalls in interpreting VaR, such as misunderstanding confidence levels (thinking 99% VaR means no losses beyond the VaR value), false sense of security if VaR isn't complemented with stress testing or tail risk measures, and the danger of using VaR as a performance metric.
Advanced Risk Measures (Extensions of VaR) for Portfolio Risk: Conditional VaR or Expected Shortfall, Incremental and Marginal VaR, and Stressed VaR
This section will provide insights into enhancements and variants of VaR that address its key weaknesses:
Conditional VaR (CVaR) or Expected Shortfall: It measures the expected loss beyond the VaR threshold, offering a better view of tail risk and satisfying the properties of a coherent risk measure.
Incremental VaR (IVaR): It evaluates the change in VaR due to the addition of a new position or asset, helping managers understand the marginal contribution to portfolio risk.
Marginal VaR (MVaR): It provides the sensitivity of VaR to small changes in position size, guiding portfolio optimization and capital allocation.
Stressed VaR (SVaR): It was introduced post-2008 financial crisis. this measure uses data from extreme market conditions to reflect how portfolios might behave under systemic stress.
The section will present formulas, practical examples, and use cases for each, especially within risk capital and regulatory frameworks.
Validating Value-at-Risk (VaR) Methodologies Through Backtesting and Stress Testing
This section will focus on validating the reliability and robustness of VaR models:
Backtesting VaR: Compares actual losses against predicted VaR values. It will include coverage tests (Kupiec test), hit ratio and exception analysis, and regulatory backtesting zones (green/yellow/red) per Basel rules.
Stress Testing: This goes beyond VaR to assess portfolio behavior in extreme but plausible scenarios. It covers historical scenario stress (2008 crisis, COVID-19 shocks), hypothetical stress scenarios, and reverse stress testing.
This section will reinforce why VaR should not be used in isolation and how model risk management frameworks incorporate validation procedures to ensure reliability in real-world use.
This introductory section sets the foundation for a structured exploration of Value-at-Risk and its role in quantitative risk management. Through this series, readers will gain a rigorous understanding of VaR computation methods, awareness of the theoretical assumptions and limitations, exposure to practical applications in risk management, and insights into extensions and model validation framework.
As financial systems become increasingly complex and intertwined, the ability to measure and manage risk quantitatively is not just desirable—it is essential. This series is intended to equip readers with the tools, methodologies, and exposure necessary to become professional risk managers.
Comments